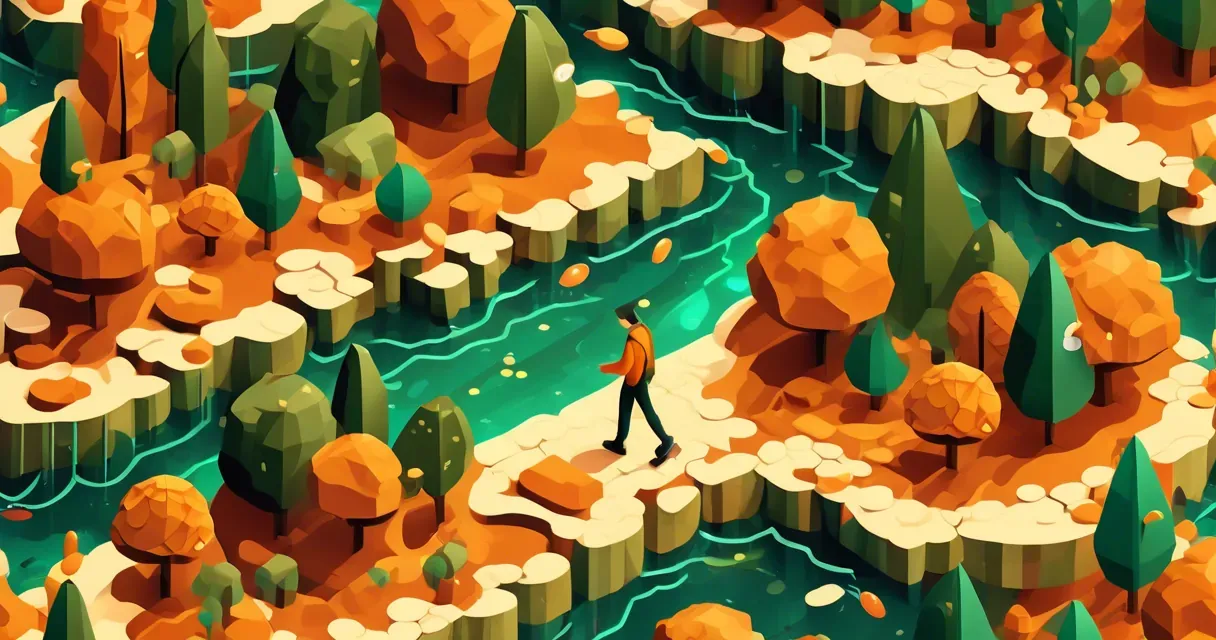
Explainable AI Enhances Malware Detection in Encrypted Traffic
/ 4 min read
Quick take - Recent research presented at the 2020 ACM Conference has introduced an explainable detection model for malware traffic that enhances cybersecurity by improving detection capabilities and transparency in AI applications, while also suggesting the need for data-driven policy development in the field.
Fast Facts
- Recent research at WiSec 2020 introduced an explainable detection model for malware traffic, enhancing cybersecurity through AI transparency.
- The model utilizes ensemble learning techniques, including Random Forest and XGBoost, to improve incident response and integrate with existing Intrusion Detection Systems (IDS).
- Key findings revealed improved detection of zero-day attacks and cross-domain malware, with Shapley Additive Explanations (SHAP) enhancing user trust in AI decisions.
- The model significantly reduced incident response times and improved threat mitigation strategies, indicating its potential for real-time network monitoring.
- Future research will focus on addressing model limitations, exploring additional explainability techniques, and fostering collaboration with law enforcement for practical applications.
In an era where cyber threats are increasingly sophisticated, understanding and mitigating these risks has never been more critical. The rapid evolution of malware tactics necessitates innovative solutions that not only enhance detection capabilities but also provide transparency in how these systems operate. Recent research points to a promising avenue: explainable artificial intelligence (XAI) integrated with advanced machine learning techniques. By leveraging ensemble learning models such as Random Forest, XGBoost, and Extremely Randomized Trees, cybersecurity experts are crafting robust frameworks aimed at improving incident response and threat mitigation.
As organizations adopt real-time network monitoring tools, the integration with intrusion detection systems (IDS) becomes paramount. These systems serve as the frontline defenders against malicious attacks by monitoring, analyzing, and responding to potential threats in real time. The findings underscore an imperative shift towards enhancing zero-day attack detection—threats that exploit vulnerabilities before they are known or patched. This proactive approach is bolstered by employing sophisticated data preparation and dataset compilation strategies that ensure the models are trained on relevant and comprehensive datasets.
The use of tree ensemble methods not only boosts the accuracy of malware detection but also facilitates cross-domain malware detection. This capability allows cybersecurity professionals to identify threats that may traverse different environments, enhancing overall security posture. Yet, the journey does not end with detection; there is a pressing need for explainability within these models. In a field where trust can be elusive, providing clear insights into how decisions are made is crucial for user acceptance and compliance with regulatory standards.
Utilizing Shapley Additive Explanations (SHAP), researchers have demonstrated how complex model decisions can be distilled into understandable narratives for stakeholders. This technique empowers users to grasp why certain actions were recommended or taken by the AI, fostering enhanced trust in automated systems. Such explainability is essential, particularly when integrating cybersecurity models with policy and security practices, where decision-making processes must be transparent and justifiable.
Despite these advancements, limitations remain evident. The complexity of encrypted traffic presents ongoing challenges for accurate detection without compromising privacy. Future research must focus on this area, exploring methodologies that balance effective monitoring with strict adherence to data protection regulations. Moreover, while current models show promise in detecting known threats, further investigation into their ability to adapt to emerging attack vectors is necessary.
The implications of this research extend beyond technical enhancements; they pave the way for data-driven policy and regulation development. As collaboration between law enforcement and cybersecurity agencies intensifies, there’s a pivotal opportunity to harness these findings to create frameworks that not only protect data but also uphold ethical considerations in technology usage.
Looking ahead, the landscape of cybersecurity will undoubtedly continue to evolve. As machine learning models become more prevalent in threat detection and response mechanisms, their continued refinement will be essential for addressing increasingly sophisticated cyber threats. The future holds potential for even greater integration of explainable AI within cybersecurity protocols, thereby enhancing user-centric security solutions that align technological capabilities with societal needs. In navigating this complex terrain, establishing a balance between innovation and accountability will prove vital for fostering a safer digital environment.