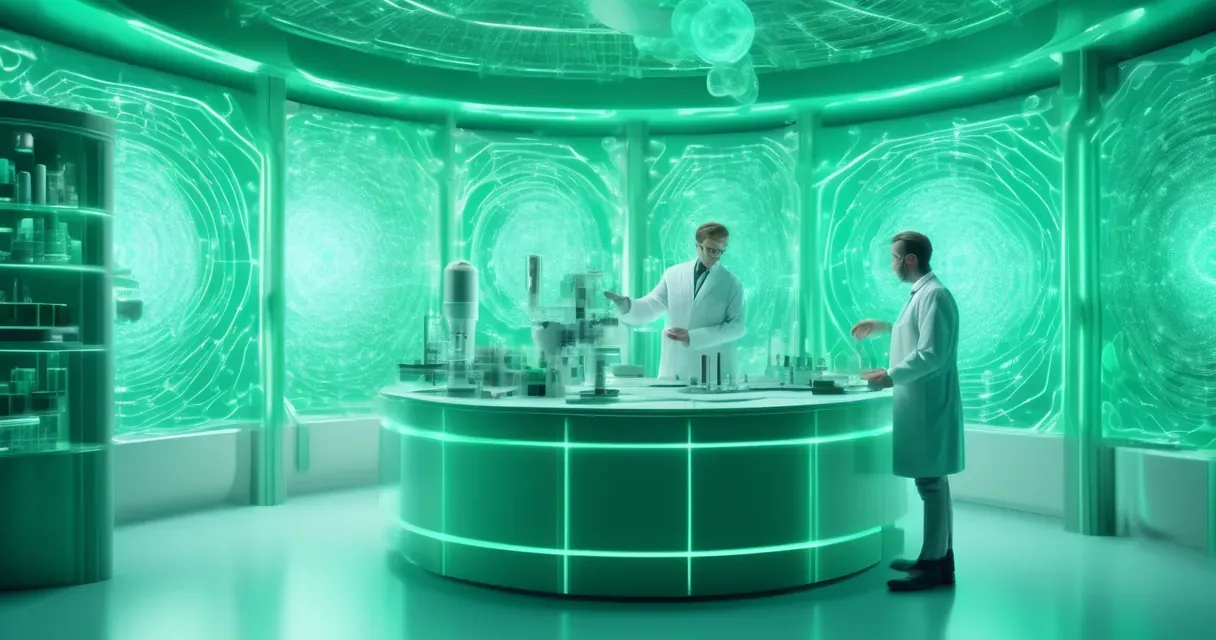
Actminer Introduces Advanced Techniques for Threat Detection
/ 4 min read
Quick take - The Actminer research presents advancements in threat hunting methodologies through semantic analysis and contextual understanding, demonstrating improved detection capabilities and operational efficiency in cybersecurity, particularly against Advanced Persistent Threats (APTs).
Fast Facts
- Innovative Threat Hunting: The Actminer system enhances threat detection through semantic analysis and contextual understanding, optimizing query graph representation for effective cybersecurity measures.
- Advanced Detection Capabilities: Actminer utilizes a heuristic search strategy based on Equivalent Semantic Transfer (EST) to identify critical nodes in cyber attacks, improving detection of Advanced Persistent Threats (APTs).
- Real-Time Integration: The system integrates with real-time threat intelligence feeds and automated incident response systems, allowing for timely updates and rapid mitigation of threats.
- Performance Improvement: Actminer outperforms the state-of-the-art system POIROT in terms of detection capabilities while maintaining operational efficiency, particularly in cloud and IoT environments.
- Future Research Directions: Suggested areas for future exploration include integrating machine learning for predictive threat hunting and expanding real-time threat intelligence sharing to enhance organizational defense strategies.
In an era where digital threats evolve at lightning speed, cybersecurity remains a pressing concern for organizations worldwide. As cybercriminals increasingly employ sophisticated tactics to breach defenses, traditional methods of threat detection are struggling to keep pace. Enter Actminer, a groundbreaking system poised to redefine how we approach threat hunting. By integrating advanced semantic analysis with real-time threat intelligence feeds, Actminer offers a fresh perspective on detecting and mitigating potential security risks, allowing businesses to stay one step ahead.
At the heart of Actminer’s methodology is its focus on causality and contextual analysis, which enhances threat detection capabilities by not just identifying malicious activity but understanding why it occurs within a specific context. This layered approach allows cybersecurity professionals to assess the relevance of detected threats more effectively. The system employs a suspicious semantic tree construction technique that identifies new candidate nodes in provenance graphs based on query graph attributes, ensuring that investigations target the most pertinent aspects of potential attacks.
Enhanced interpretability and visualization tools play a crucial role in this process. Rather than overwhelming analysts with raw data, Actminer transforms complex datasets into actionable insights, enabling them to make informed decisions quickly. The integration of automated incident response systems further streamlines operations by facilitating swift actions against identified threats, thereby reducing the window of vulnerability.
One of Actminer’s notable strengths lies in its cross-platform adaptability, allowing it to function effectively across diverse environments, including cloud and Internet of Things (IoT) ecosystems. This flexibility is vital as organizations increasingly migrate their operations to the cloud and adopt IoT technologies, exposing themselves to new vulnerabilities. Yet, as promising as Actminer appears, there are limitations to consider—particularly concerning its robustness when faced with benign datasets. Understanding how the system can avoid false alerts while maintaining high detection accuracy remains a critical area for future investigation.
The research also underscores the importance of real-time threat intelligence sharing, optimizing query graph representations for efficient threat hunting. By enhancing user behavior analytics (UBA) and integrating cyber threat intelligence (CTI), Actminer can significantly improve its predictive capabilities. The incorporation of machine learning algorithms further amplifies its effectiveness in anticipating potential threats before they materialize.
A performance comparison reveals that Actminer outperforms state-of-the-art systems like POIROT in detecting Advanced Persistent Threats (APTs), particularly in terms of runtime overhead and timeliness. This performance boost highlights the system’s operational efficiency and positions it as a formidable tool in the cybersecurity arsenal.
As we look toward the future, the implications of these findings are profound. Advanced systems like Actminer are not simply incremental upgrades; they represent a paradigm shift in how organizations can protect themselves against ever-evolving cyber threats. With continued focus on refining techniques such as incremental aligning modules and heuristic search strategies based on equivalent semantic transfer, we may soon witness a transformation in the efficacy and efficiency of threat detection methodologies.
In an age where data breaches can lead to catastrophic financial and reputational damage, investing in robust cybersecurity frameworks is no longer optional but essential. Actminer serves as a beacon of hope for organizations eager to bolster their defenses against an unpredictable digital landscape, paving the way for innovative solutions that will shape the future of cybersecurity practice.
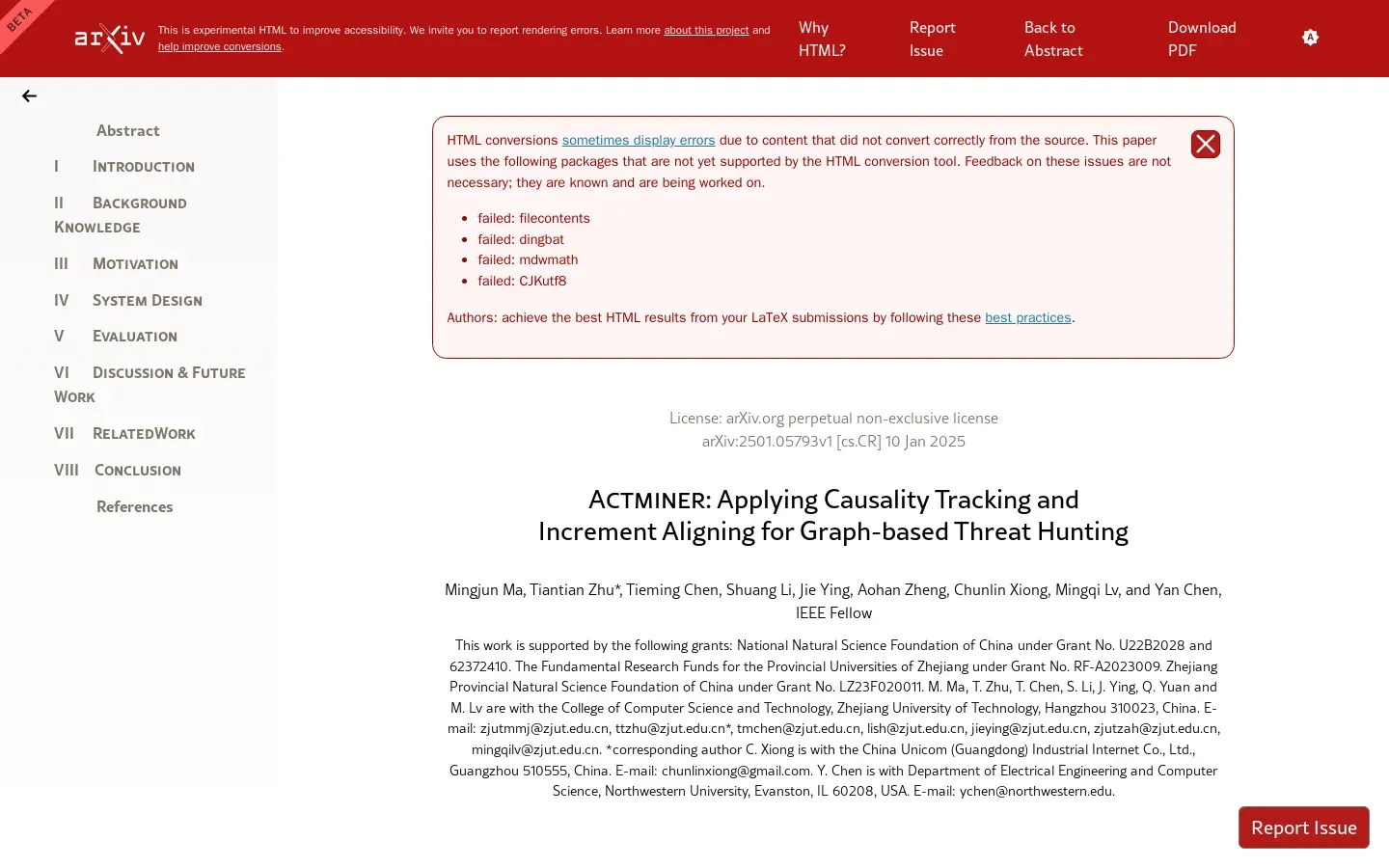