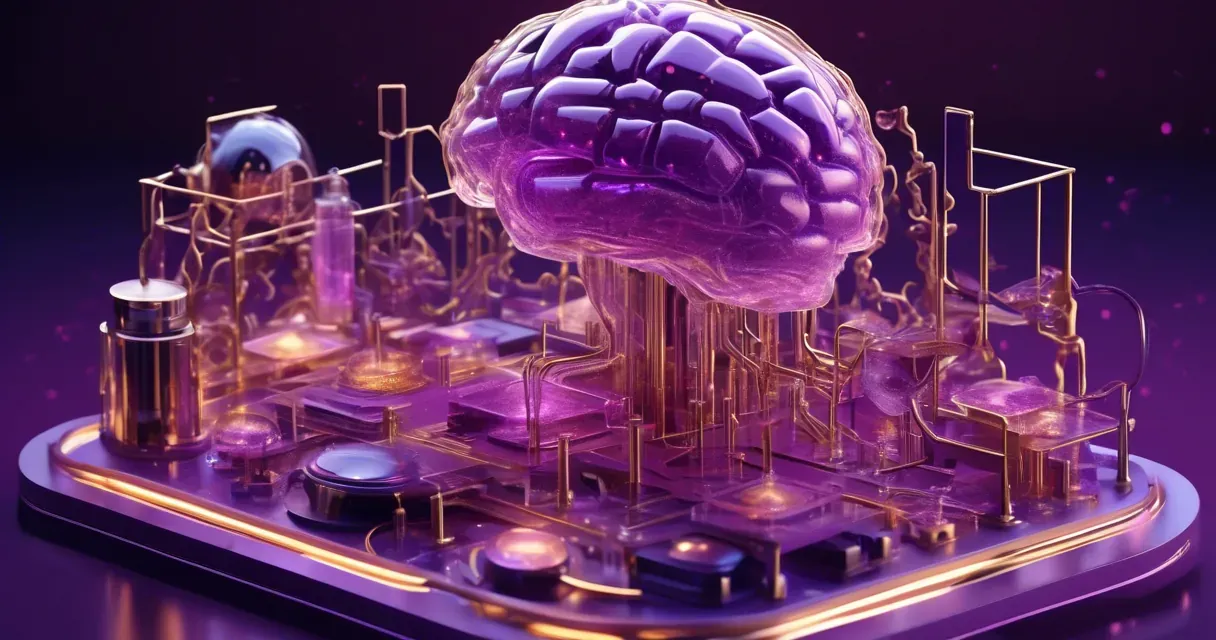
Research Introduces VisUnpack Framework for Malware Detection
/ 4 min read
Quick take - Recent research has introduced the VisUnpack framework, which enhances malware detection and classification by analyzing malware in smaller units and employing advanced methodologies, thereby addressing current challenges and paving the way for future advancements in cybersecurity.
Fast Facts
- The VisUnpack framework enhances malware detection by analyzing packed malware samples in smaller units, improving classification efficiency and scalability.
- Key methodologies include advanced static analysis, adaptive learning algorithms, and the use of the VGG11 neural network for machine learning classification.
- The research highlights the critical role of unpacking malware for accurate detection and emphasizes the need for integrating advanced analytical techniques into existing cybersecurity frameworks.
- Strengths of the study include the development of lightweight security solutions and efficient handling of large datasets, while limitations focus on the challenges posed by packing techniques.
- Future directions involve collaboration with threat intelligence platforms, development of advanced analysis tools, and a shift towards context-aware approaches to adapt to evolving malware threats.
In the fast-paced realm of cybersecurity, where threats evolve with each click and keystroke, malware detection remains a critical frontier. The rise of sophisticated packing techniques has left traditional detection methods struggling to keep pace, leading to an urgent need for innovation in this domain. Recent research highlights the importance of advanced analytical methodologies, like the VisUnpack framework, which offers a promising approach to tackling these challenges. By focusing on smaller, manageable units—known as basic blocks—this framework enables a more efficient and scalable analysis process. This efficiency is vital as attackers increasingly leverage packing to obscure their malicious intents, rendering conventional methods less effective.
One of the standout findings of this research underscores the impact of packing on classification accuracy. When malware samples are packed, they can significantly hinder accurate classification efforts, making unpacking an essential step for effective threat assessment. The VisUnpack framework directly addresses this issue by implementing Local Similarity Descriptors (LSD) that facilitate more precise unpacking and classification strategies. This method not only enhances detection rates but also optimizes resource consumption—a crucial factor in the age of real-time malware analysis.
The study further delves into the importance of context-sensitive analysis, advocating for methodologies that adapt dynamically to the evolving nature of malware threats. This shift towards adaptive learning is pivotal, allowing systems to keep pace with emerging attack vectors while maintaining high levels of detection accuracy. By integrating advanced analytical techniques such as Binned Log-Polar Representation, which processes information in a way that preserves essential data while reducing noise, cybersecurity frameworks can bolster their defenses against increasingly intricate attack strategies.
A noteworthy aspect of the research is its call for improved collaboration with threat intelligence platforms. Such partnerships could enhance the effectiveness of existing security measures, aiding organizations in staying ahead of potential breaches. By utilizing tools like VirusTotal and radare2 alongside the VisUnpack framework, cybersecurity teams can achieve a more rounded and robust defense posture.
As organizations seek to fortify their cybersecurity infrastructures, investing in training and education becomes imperative. Equipping teams with knowledge about emerging malware trends and unpacking techniques will serve as a significant advantage in thwarting attacks before they escalate. Furthermore, real-time malware detection systems must evolve to integrate these findings seamlessly into their protocols, ensuring that as new threats emerge, defenses remain agile and informed.
Looking ahead, the implications of these findings are profound. The development of lightweight mobile security solutions stands out as a future direction worth pursuing. As mobile devices become prime targets for cybercriminals, ensuring that security measures are both effective and efficient will be paramount. Additionally, there’s a clear need for ongoing research into packing techniques and their impact on detection efficacy; understanding these dynamics could lead to breakthrough advancements in malware classification.
In conclusion, as cyber threats continue to morph and multiply, it is evident that innovation in malware detection methodologies is not just beneficial but necessary. The integration of frameworks like VisUnpack with continuous adaptability and collaborative efforts will empower security professionals to combat malware more effectively than ever before. The journey toward enhanced cybersecurity is ongoing, but with these advancements, we can anticipate a future where our defenses are not only reactive but proactively resilient against the evolving landscape of cyber threats.