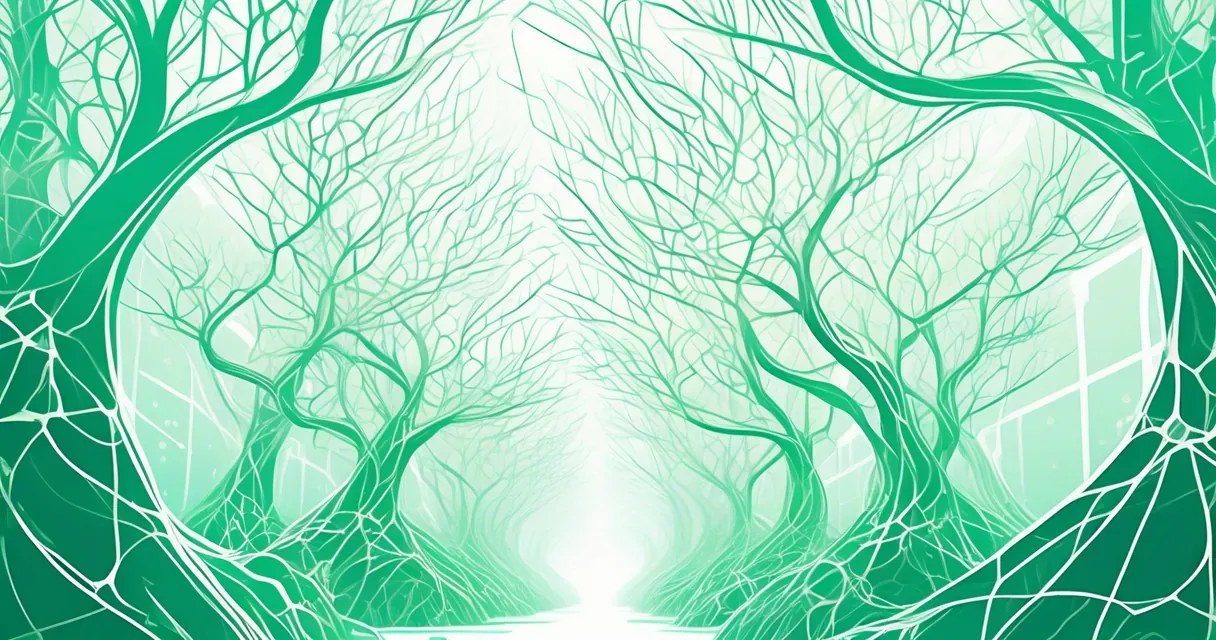
ByzSFL Enhances Secure Federated Learning with Zero-Knowledge Proofs
/ 4 min read
Quick take - Recent research on the DuoAgg protocol highlights its potential to enhance the security and efficiency of federated learning by integrating Byzantine-robust aggregation techniques and zero-knowledge proofs, thereby improving resilience against malicious attacks while maintaining data privacy.
Fast Facts
- DuoAgg Framework: Integrates Byzantine-robust aggregation and zero-knowledge proofs to enhance security and efficiency in federated learning, addressing vulnerabilities in centralized architectures.
- Byzantine Resilience: The framework effectively withstands adversarial attacks, ensuring the integrity of model updates through robust aggregation techniques.
- Real-Time Threat Detection: Utilizes zero-knowledge proofs for immediate input validation, allowing quick identification and response to potential security threats.
- Cross-Domain Versatility: Applicable in various sectors, including healthcare and finance, demonstrating its potential for secure data collaboration.
- Future Research Directions: Emphasizes the need for practical implementation strategies, integration with smart city infrastructures, and scalability for larger datasets in secure federated learning.
In an era where the convergence of technology and security is more critical than ever, researchers are tirelessly working to fortify our digital infrastructures. The advent of federated learning (FL) has stirred excitement in cybersecurity circles, promising a new frontier in collaborative artificial intelligence while maintaining stringent data privacy standards. One standout development in this realm is the DuoAgg framework, a sophisticated protocol designed to tackle some of the most pressing challenges associated with secure federated learning. By distributing tasks between two independent servers, DuoAgg not only enhances efficiency but also bolsters security against potential breaches that could compromise sensitive data.
The backbone of this innovative approach lies in its real-time threat detection and response capabilities. In today’s fast-paced digital landscape, the ability to identify and mitigate threats as they arise can mean the difference between a minor inconvenience and a catastrophic data breach. This capability is complemented by utilization of Byzantine-robust aggregation techniques, which ensure that even if certain nodes within a network are compromised, the overall integrity of the system remains intact. Such resilience is paramount in environments where trust is tenuous at best.
The research surrounding these frameworks also touches on homomorphic encryption (HE), a cryptographic method that allows computations on encrypted data without needing to decrypt it first. This technique ensures that sensitive information remains confidential during processing, making it particularly advantageous for sectors such as healthcare, where patient data privacy is non-negotiable. As healthcare data collaboration continues to grow, integrating these advanced encryption methods will be essential for safeguarding personal information while still enabling valuable insights gleaned from shared datasets.
Equally compelling are zero-knowledge proofs (ZKP)—a cryptographic technique that allows one party to prove to another that a statement is true without revealing any additional information. In the context of distributed server architectures used in federated learning, ZKPs can enhance input validation processes, ensuring that only legitimate data contributes to model training. This not only strengthens data integrity but also supports cross-domain applications, making federated learning viable in various industries beyond traditional tech sectors.
Nevertheless, despite these advancements, there remain critical limitations. The implementation of DuoAgg raises questions about scalability and operational complexity when rolling out across diverse systems. Furthermore, while the integration of blockchain technology into smart city infrastructures presents exciting possibilities for decentralized machine learning platforms, the real-world implications of such frameworks require further investigation. How can we ensure that all components interact seamlessly without introducing new vulnerabilities?
As we examine the implications for cybersecurity through the lens of these findings, it becomes clear that adopting robust technologies like Byzantine resilience and enhanced aggregation methods holds significant promise for improving not just security measures but also overall computational efficiency. Financial fraud detection systems stand to benefit greatly from these innovations, as they rely heavily on rapid anomaly detection—a task made easier through federated learning’s collaborative nature.
Looking ahead, the future appears bright yet demanding. The ongoing evolution of federated learning frameworks must be accompanied by rigorous testing and adaptation to emerging threats. As organizations increasingly leverage AI-driven solutions for cybersecurity, they must remain vigilant about maintaining privacy and integrity within their systems. The path forward will undoubtedly involve continuous refinement of models like DuoAgg and exploration into novel cryptographic techniques that push the boundaries of what secure collaborations can achieve in an interconnected world. As we embrace these technologies, it is imperative that we foster a culture of vigilance and adaptability to safeguard our digital futures against an ever-evolving landscape of cyber threats.