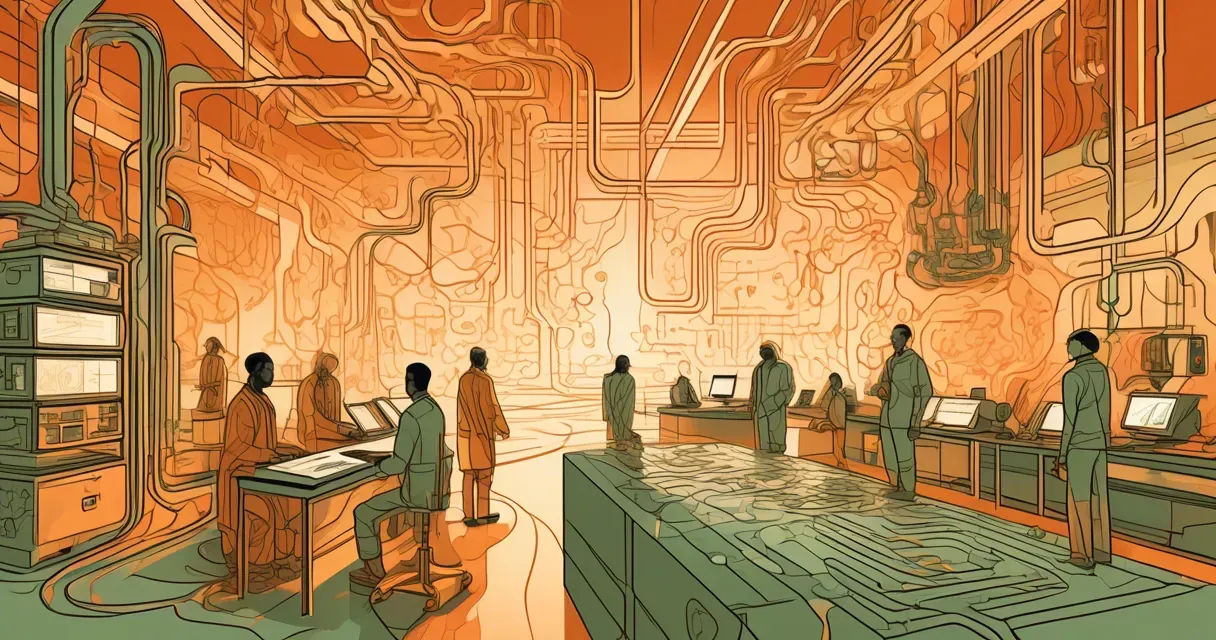
Generative AI Tools in Penetration Testing: A Comparative Study
/ 4 min read
Quick take - A recent study highlights the potential of Generative AI tools to enhance penetration testing and vulnerability exploitation in cybersecurity, while also emphasizing the need for ethical oversight and human intervention in their application.
Fast Facts
- Generative AI Integration: The study explores the use of GenAI tools like GPT-4 and Microsoft Copilot to enhance penetration testing workflows, improving vulnerability identification and exploitation processes.
- Key Findings: AI tools significantly improve vulnerability identification accuracy, automate threat intelligence generation, and enhance incident response automation, leading to more efficient cybersecurity practices.
- Strengths and Applications: The research highlights potential applications for AI in customizing commands for pentesting, developing AI-driven security tools, and creating personalized security awareness training programs.
- Ethical Considerations: The study emphasizes the need for human oversight in AI-driven cybersecurity processes and warns against the potential for malicious use of advanced AI tools.
- Future Directions: Recommendations include further integration of AI in incident response, development of AI-driven training environments, and establishment of standards for ethical AI use in penetration testing.
In an era where digital threats are evolving at lightning speed, the intersection of cybersecurity and artificial intelligence (AI) has emerged as a pivotal area of research. As organizations scramble to fortify their defenses against increasingly sophisticated attacks, the deployment of Generative AI tools in penetration testing is not just a trend but a necessity. This dynamic duo promises enhanced efficiency and effectiveness in identifying vulnerabilities, automating exploitation processes, and streamlining incident response protocols. Nevertheless, as with any powerful technology, it comes with its own set of limitations and ethical considerations that cannot be overlooked.
The recent research into employing Generative AI tools like GPT-4, Claude Opus, and Microsoft Copilot demonstrates a structured methodology for conducting penetration tests. This involves meticulous steps ranging from vulnerability identification to the critical phases of exploitation and post-exploitation activities. At the heart of this methodology lies the capability to generate context-specific commands, allowing cybersecurity professionals to navigate complex environments with greater agility. Tools such as ChatGPT facilitate objective setting post-exploitation by producing actionable insights like enumeration and privilege escalation strategies—essential for maintaining momentum during assessments.
As organizations increasingly rely on automated frameworks for exploitation, they are witnessing a significant uptick in efficiency. The integration of tools like Certipy and Impacket aids in vulnerability analysis while enhancing threat intelligence generation. These advancements not only expedite the penetration testing process but also pave the way for more personalized security awareness training tailored to specific organizational needs. By customizing command outputs based on the unique context of each engagement, teams can better prepare for potential exploits.
Yet, while these AI-driven innovations present substantial strengths, they are not without their pitfalls. The limitations inherent in generative AI applications raise pressing concerns about human oversight and ethical implications in cybersecurity practices. The potential for malicious use of these technologies looms large, necessitating rigorous scrutiny and governance frameworks to prevent their exploitation by adversaries. Additionally, while automation can enhance incident response capabilities, it should not replace the irreplaceable intuition and judgment that skilled cybersecurity professionals bring to the table.
Looking ahead, the implications for training and skill development within the cybersecurity workforce are profound. Future research might prioritize enhancing customization features of AI tools to ensure they can deliver even more detailed and contextually relevant commands for pentesting tasks. Furthermore, as organizations embrace these technologies, there will be a growing need for comprehensive training programs that equip professionals with both technical skills and ethical decision-making frameworks.
In conclusion, while Generative AI tools hold immense promise for revolutionizing penetration testing and vulnerability management in cybersecurity, their effective implementation requires a balanced approach that prioritizes human insight alongside automated efficiency. As we navigate this rapidly changing landscape, it is crucial to foster an environment where innovation thrives under careful stewardship—a commitment that will ultimately shape the future of cybersecurity in an increasingly complex digital world.