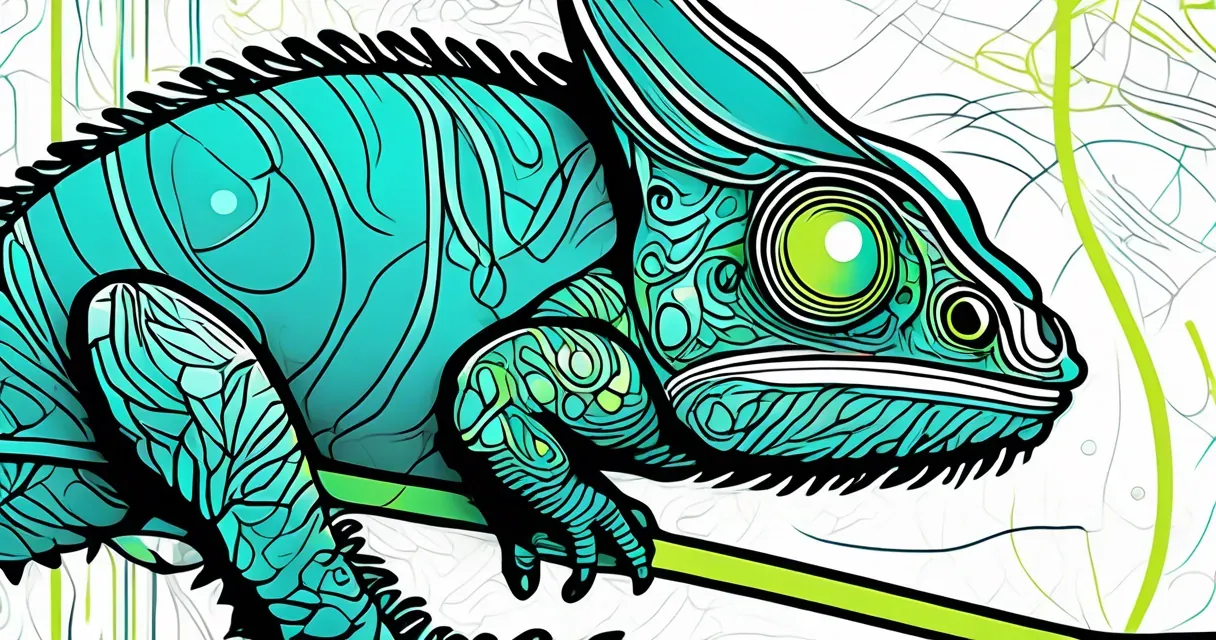
New Method Enhances Intrusion Detection System Adaptability
/ 4 min read
Quick take - Researchers have introduced a novel continual learning method called Strategic Sample Selection and Forgetting (SSF) to improve the adaptability and efficiency of Intrusion Detection Systems (IDS) in response to evolving cyber threats, particularly in Internet of Things (IoT) and edge computing environments.
Fast Facts
- Researchers introduced the Strategic Sample Selection and Forgetting (SSF) method to enhance Intrusion Detection Systems (IDS) in response to evolving cyber threats, particularly in IoT and edge computing environments.
- The SSF method effectively manages concept drift by strategically selecting representative samples for model training, optimizing resource usage, and conserving labeling resources.
- Integration of regularization-based methods like Learning without Forgetting (LwF) and memory-based techniques such as Experience Replay (ER) improves model performance and resilience against threats.
- The SSF method can be integrated with automated incident response systems, enhancing the speed and accuracy of threat detection while ensuring user-centric customization and explainability.
- Future research directions include real-time cyber threat intelligence sharing and advanced model fine-tuning techniques to further improve the adaptability of IDS against emerging threats.
In the ever-evolving landscape of cybersecurity, where threats grow more sophisticated by the day, researchers are tirelessly innovating to stay one step ahead. Recent findings from a groundbreaking study on continual learning methods for Intrusion Detection Systems (IDS) reveal transformative approaches that promise not just to enhance security protocols but also to redefine how we think about data management in a world rife with cyber threats. This study introduces a Memory Buffer Update strategy characterized by strategic forgetting, which could be pivotal for organizations grappling with limited labeling resources and the need for adaptability.
At the core of this research lies the concept of Common Distribution Representation, which facilitates the selection of older samples that best represent the evolving data landscape. This phase significantly reduces the demand for extensive labeling efforts, allowing cybersecurity professionals to harness previously labeled data efficiently. By leveraging techniques like Learning without Forgetting (LwF), systems can maintain performance levels while adapting to new data trends without losing valuable information from past experiences.
The study also highlights a critical component: user-centric customization and explainability. In an industry where transparency is paramount, being able to articulate how an IDS reaches its conclusions can foster trust among users and stakeholders alike. The integration of user feedback into these systems ensures that they remain relevant and effective, tailored to specific organizational needs while still upholding robust security measures.
Nevertheless, challenges persist, particularly regarding strategic sample selection. This entails identifying which samples to retain or forget as data evolves — a process crucial for maintaining model accuracy amidst concept drift. Techniques like the Kolmogorov-Smirnov (K-S) Test offer methodologies for assessing shifts in data distributions, allowing systems to detect when models may be at risk of obsolescence. This proactive approach is essential for ensuring that defenses remain resilient against emerging threats.
Furthermore, the implications of this research extend beyond traditional domains; it opens avenues for cross-domain adaptation, enabling models trained in one environment to apply their learnings in another. For instance, as IoT devices proliferate, adaptive security measures become paramount. The ability to swiftly train models on representative samples ensures that even these vulnerable endpoints benefit from cutting-edge intrusion detection capabilities.
Among the recommended tools discussed, the Strategic Sample Selection Algorithm stands out as a vital component of this methodology, enabling real-time adaptability without overwhelming resources. Coupled with a robust strategic forgetting mechanism, organizations can ensure that their IDS remains agile and responsive in dynamic environments. This synergy not only enhances resilience but also aligns with current trends toward real-time cyber threat intelligence sharing, making systems smarter and more integrated.
Looking forward, as cybersecurity threats continue to evolve, so too must our strategies for countering them. The integration of these innovative methodologies into automated incident response systems will likely play a crucial role in shaping future defenses. By embracing memory-based methods such as Experience Replay (ER) and analyzing divergence through Kullback-Leibler metrics, cybersecurity frameworks can achieve unprecedented levels of sophistication and responsiveness.
In conclusion, while the path ahead is fraught with challenges, the strategic advancements outlined in this research signal a promising shift towards more resilient cybersecurity paradigms. As these methods find their way into practical applications across industries — especially within IoT and edge computing environments — we may well be entering a new era of security where adaptability is not just beneficial but essential for survival in the digital age.
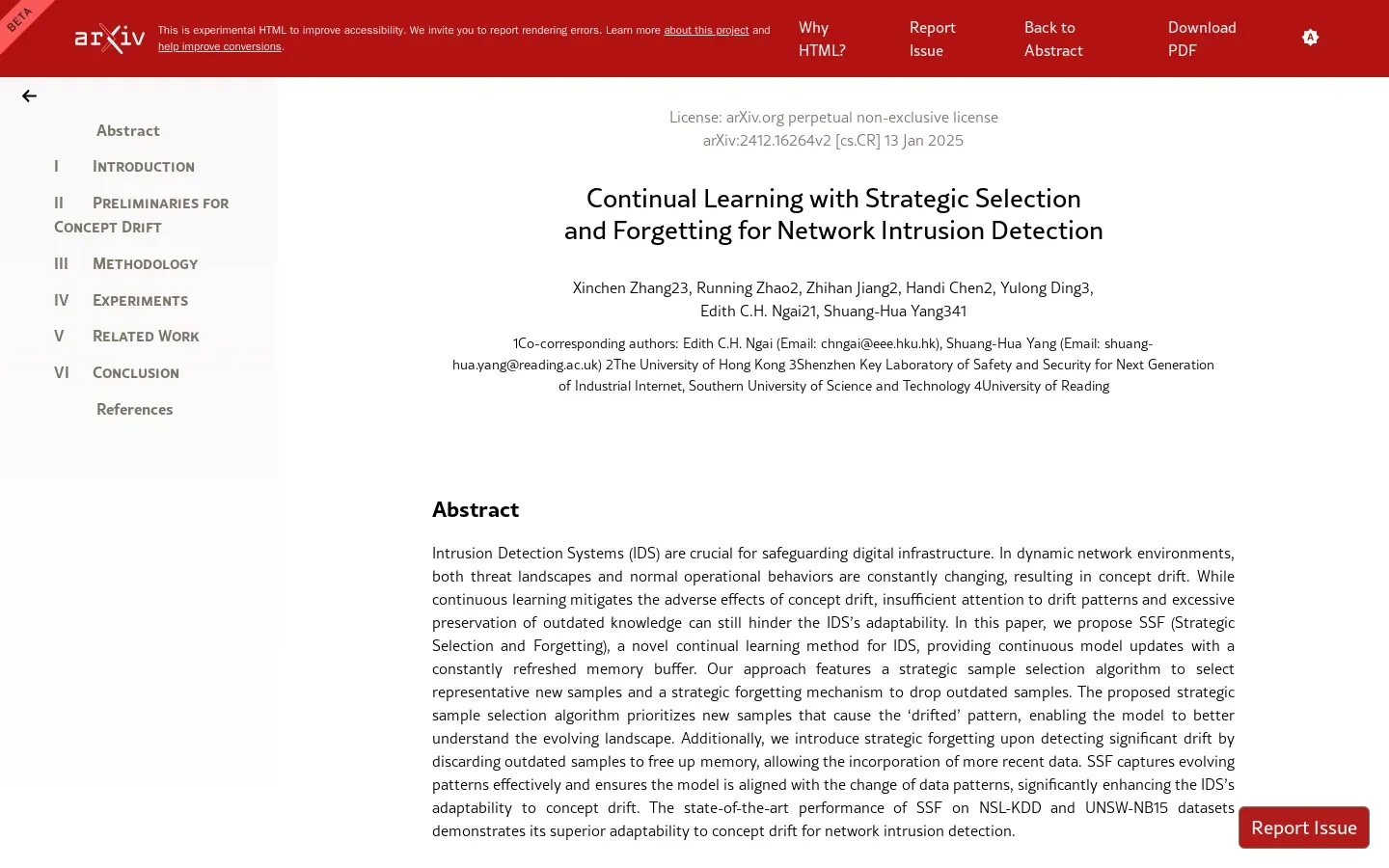