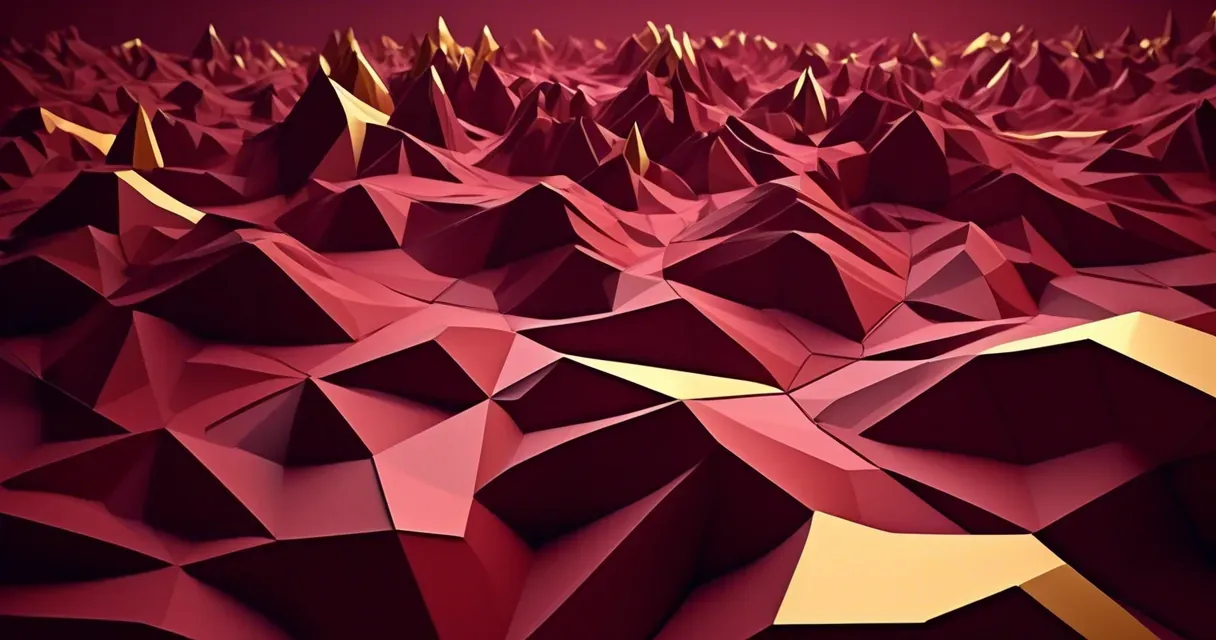
TFLAG Framework Enhances APT Detection Using Graph Neural Networks
/ 4 min read
Quick take - The TFLAG framework, introduced in a recent study, enhances the detection of Advanced Persistent Threats (APTs) by integrating temporal features with deviation networks, utilizing Graph Neural Networks and self-supervised learning to improve real-time monitoring and incident response in cybersecurity.
Fast Facts
- The TFLAG (Temporal Framework for Anomaly Graphs) framework enhances detection of Advanced Persistent Threats (APTs) by integrating temporal features with deviation networks, addressing gaps in current methodologies.
- Utilizing Graph Neural Networks (GNNs) and self-supervised learning, TFLAG minimizes reliance on extensive labeled datasets, adapting to evolving cyber threats.
- The framework significantly reduces false positives and improves anomaly detection by analyzing threat behaviors over time, making it applicable across various sectors, including finance and healthcare.
- Key tools include dynamic temporal graph construction and deviation networks, which facilitate timely responses to potential threats and enhance predictive capabilities through integration with threat intelligence platforms.
- Future research aims to refine TFLAG by incorporating additional machine learning techniques and adapting to the evolving landscape of cyber threats for improved real-time monitoring and incident response.
In an era where cyber threats loom larger than ever, the need for robust and adaptive cybersecurity solutions is paramount. As organizations increasingly rely on digital infrastructures, the sophistication of attacks, particularly Advanced Persistent Threats (APTs), poses significant challenges. Recent research has illuminated innovative frameworks and methodologies aimed at bolstering our defenses against these insidious intrusions. One such groundbreaking framework is TFLAG, which stands at the forefront of enhancing detection capabilities through a combination of advanced technologies.
The TFLAG framework introduces a multifaceted approach to APT detection by leveraging provenance graphs to map out the intricate relationships between entities within complex systems. This capability is crucial as it allows for dynamic temporal graph construction, enabling real-time updates that reflect the evolving nature of threats. The integration of temporal features into deviation networks enhances the system’s ability to detect anomalies that would otherwise go unnoticed in static models. This adaptability is vital; cyber attackers constantly refine their strategies, and any lag in detection can lead to catastrophic breaches.
A notable strength of this research lies in its incorporation of self-supervised learning mechanisms. By harnessing large volumes of unlabeled data, these systems can train themselves to recognize patterns indicative of malicious activity. This paradigm shift from traditional supervised learning not only reduces the reliance on extensive labeled datasets but also empowers the model to continuously learn and adapt as new threats emerge. Consequently, this method significantly diminishes false positives—a common pain point that often leads to alert fatigue among security teams.
In addition, graph neural networks (GNNs) play a pivotal role in enhancing the framework’s analytical prowess. GNNs are designed to process data structured as graphs, making them ideally suited for analyzing complex interactions within network environments. Their application within TFLAG facilitates a more nuanced understanding of how threats propagate through systems, allowing security measures to be deployed preemptively rather than reactively.
The implications extend beyond immediate detection capabilities; TFLAG also integrates seamlessly with threat intelligence platforms. This synergy enables organizations to leverage external insights alongside internal monitoring efforts, creating a comprehensive threat landscape overview. Such collaboration fosters a proactive security posture that is essential in today’s hyperconnected world.
While promising, this research does not shy away from addressing limitations and areas for further investigation. For instance, while TFLAG shows remarkable potential in anomaly detection and evaluation, its efficacy in diverse operational environments—such as industrial control systems (ICS)—remains to be thoroughly validated. Additionally, dynamic adaptation to evolving threats requires continuous refinement of the algorithms powering these models; ongoing research must focus on ensuring that they remain relevant amidst shifting attack vectors.
As we look ahead, the potential for frameworks like TFLAG to revolutionize cybersecurity cannot be overstated. With each advancement in technology comes an opportunity to stay one step ahead of cyber adversaries. The fusion of self-supervised learning with sophisticated anomaly detection techniques heralds a new era of cybersecurity resilience, one where organizations can navigate an increasingly treacherous landscape with confidence. The journey towards fortified defenses is ongoing, but with tools like TFLAG leading the charge, there is hope for a future where APTs are met with swift and effective countermeasures.
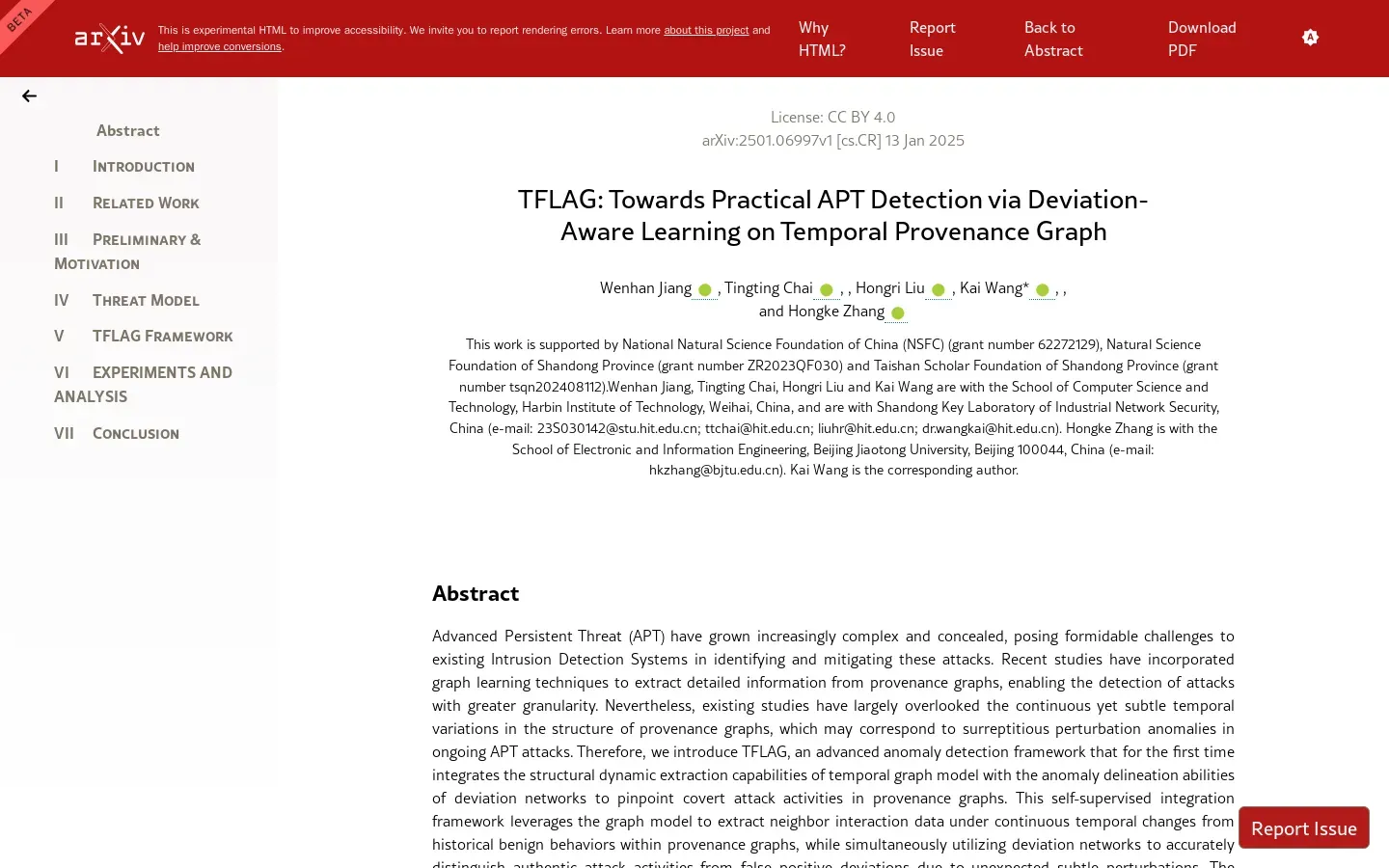