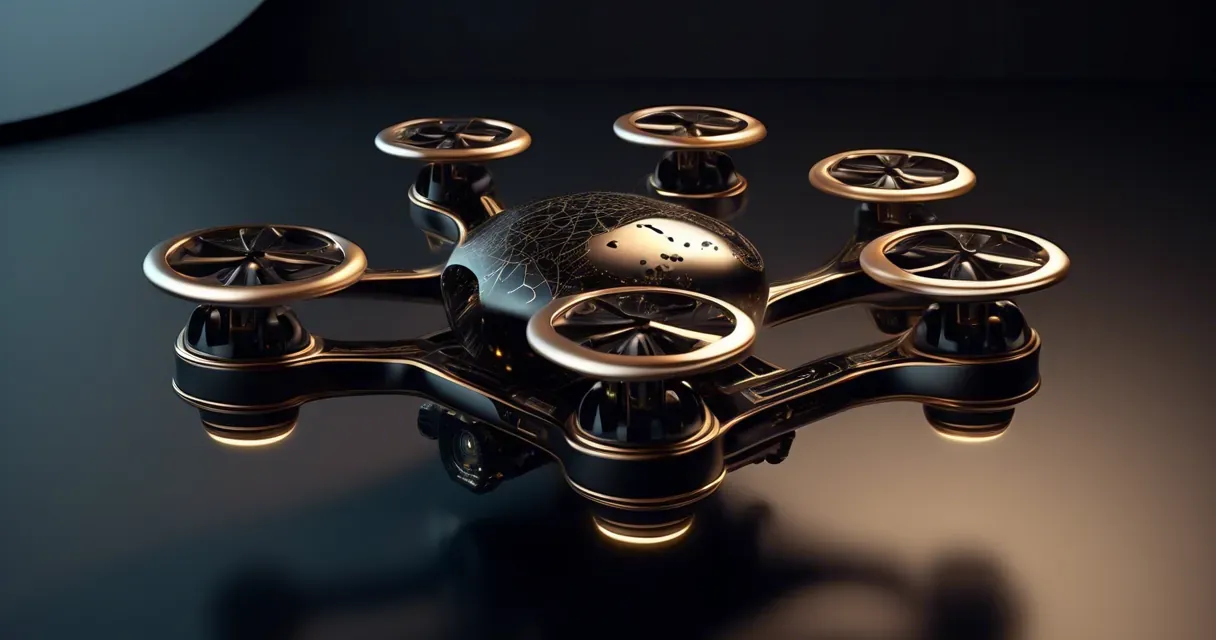
QUADFormer Framework Enhances GPS Spoofing Detection for Quadrotors
/ 4 min read
Quick take - The QUADFormer framework has been developed to enhance the cybersecurity of quadrotor UAVs by improving threat detection, enabling real-time responses, and integrating multi-modal sensor data, while also addressing regulatory compliance and future scalability challenges.
Fast Facts
- QUADFormer Framework: A new approach to enhance cybersecurity for quadrotor UAVs, focusing on detecting and mitigating cyber threats effectively.
- Superior Detection: Utilizes a transformer-based mechanism for improved threat detection compared to traditional methods, with real-time response capabilities to minimize damage.
- Adaptive Learning: Continuously learns from new data to adapt detection algorithms, ensuring resilience against evolving cyber threats.
- Multi-Modal Sensor Fusion: Integrates data from various sensors to enhance situational awareness and accuracy in threat detection.
- Future Directions: Plans to expand QUADFormer with advanced machine learning techniques and standardized protocols for broader industry application and collaboration.
In an age where drones play a pivotal role in both commercial and military operations, ensuring their security from cyber threats has become paramount. Quadrotor UAVs (unmanned aerial vehicles), with their increasing integration into critical infrastructures, have emerged as potential targets for sophisticated cyber attacks. The QUADFormer framework represents a significant leap forward in this arena, leveraging advanced technologies to bolster the resilience of these aerial systems against evolving threats. This innovative approach combines transformer-based attack detection with multi-modal sensor fusion, enabling a robust mechanism to identify and counteract malicious activities in real-time.
One of the standout features of QUADFormer is its resilient state estimation module, which utilizes an Extended Kalman Filter (EKF) for residue generation. This sophisticated method enhances the accuracy of threat detection by continuously updating the state of the UAV and discerning anomalies that may indicate an impending cyber intrusion. As the landscape of cybersecurity becomes increasingly complex, adaptive learning and continuous improvement are crucial. QUADFormer embodies this principle, allowing systems to learn from past incidents and improve their defenses autonomously over time.
The need for real-time response mechanisms cannot be overstated, especially when considering the rapid pace at which cyber attacks can manifest. QUADFormer addresses this challenge head-on by enabling instantaneous threat intelligence sharing among multiple platforms. This collaborative aspect not only enhances the UAV’s situational awareness but also fosters a community-centric defense model that augments individual system capabilities through shared insights.
Yet, as promising as these developments are, they are not without limitations. The research underscores a need for regulatory compliance and standardization across the board. Without a cohesive framework governing how these technologies operate, there remains a risk of fragmented implementations that could undermine their overall effectiveness. Furthermore, while QUADFormer showcases enhanced detection capabilities, rigorous testing through comprehensive evaluations is essential to ascertain its performance relative to existing state-of-the-art techniques, both traditional and machine learning-based.
The interdisciplinary approach adopted in developing QUADFormer highlights another critical aspect of modern cybersecurity—collaboration across various fields. By integrating insights from engineering, computer science, and operational management, QUADFormer emerges not just as a technological solution but as part of a broader strategy to enhance resilience in cyber-physical systems. This holistic view is paramount; as threats evolve, so too must our strategies for defense.
The emphasis on integrating other cyber-physical systems within the QUADFormer framework opens avenues for more extensive applications beyond UAVs. Such integration could potentially lead to unified defense mechanisms across diverse platforms that share similar vulnerabilities. Moreover, the application of real-time threat intelligence sharing ensures that lessons learned from one domain can inform practices in another, creating a dynamic ecosystem of cybersecurity vigilance.
As we look to the future, it will be critical for researchers and practitioners alike to delve deeper into areas flagged by current studies—the quest for improved adaptive learning mechanisms and more resilient frameworks will shape how effectively we can protect our digital and physical infrastructures from cyber threats. With technological advancements accelerating at an unprecedented pace, staying ahead in this game requires not only innovation but also collaboration and adherence to regulatory standards that ensure safety across all domains. The journey towards creating truly resilient cyber-physical systems is just beginning, offering vast potential yet requiring concerted effort from all stakeholders involved.