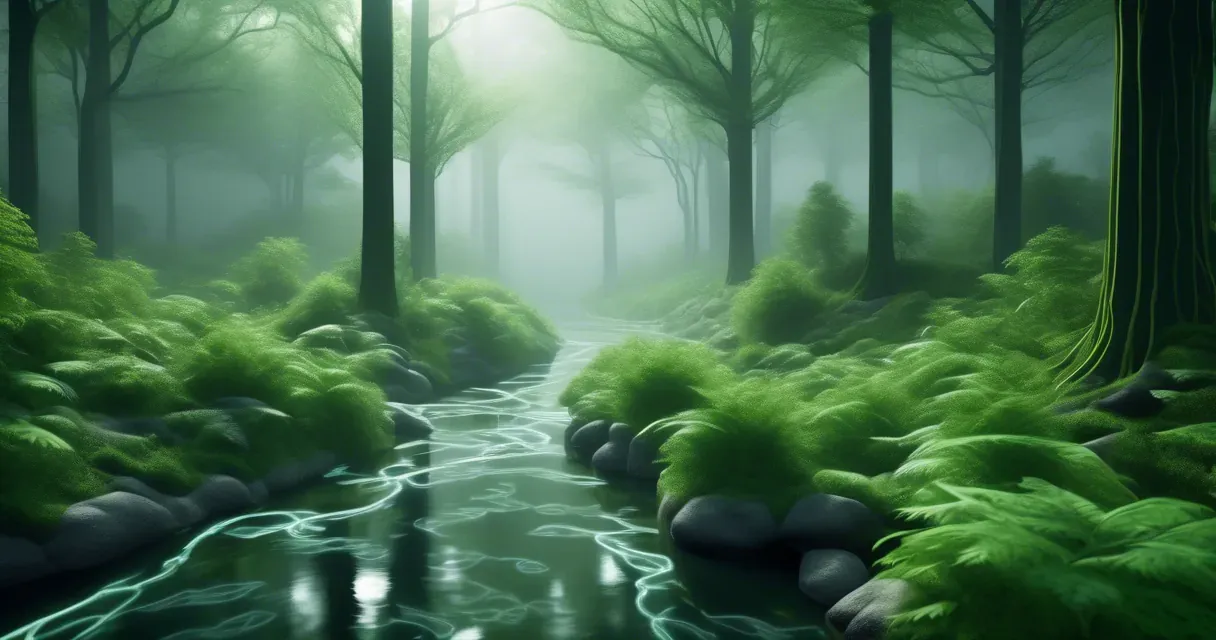
FlowID Framework Enhances Network Traffic Detection Capabilities
/ 4 min read
Quick take - The FlowID framework, developed by researchers led by Jiajun Zhou, presents a multi-view correlation-aware approach to enhance network traffic detection and improve intrusion detection systems, particularly in the context of encrypted traffic and IoT environments, while utilizing advanced machine learning techniques.
Fast Facts
-
Introduction of FlowID Framework: Developed by Jiajun Zhou et al., FlowID is a multi-view correlation-aware approach aimed at enhancing network traffic detection and improving intrusion detection systems (IDS), particularly in the context of encrypted traffic.
-
Key Methodologies: The research employs multi-view traffic feature extraction, flow hypergraph construction, semi-supervised learning, and adaptive security policies using reinforcement learning to enhance detection accuracy.
-
Significant Findings: FlowID demonstrated real-time threat detection in IoT environments, improved malware detection and classification, and facilitated automated threat intelligence sharing among organizations.
-
Technological Support: The framework utilizes advanced tools like Graph Neural Networks (GNNs) and Long Short-Term Memory (LSTM) networks to enhance network traffic representation and time-series analysis.
-
Future Directions: The study emphasizes the need for automated threat intelligence sharing, targeted IoT security management, and exploring cross-domain applications to strengthen cybersecurity measures against evolving threats.
In the ever-evolving landscape of cybersecurity, where threats loom larger and more sophisticated by the day, innovative frameworks and methodologies are paramount. The recent research led by Jiajun Zhou et al. on the FlowID framework exemplifies this forward-thinking approach, focusing on multi-view correlation-aware techniques for enhanced network traffic detection. As organizations increasingly rely on digital infrastructure, the need for robust intrusion detection systems (IDS) has never been more critical. The research highlights not only the strengths of FlowID but also its potential applications in real-world cybersecurity scenarios.
One of the standout features of FlowID lies in its ability to automate threat intelligence sharing, which addresses a significant gap in collaborative cybersecurity efforts. By facilitating real-time information exchange among organizations, FlowID empowers defenders to stay one step ahead of cyber adversaries. This capability is particularly crucial given the increasing complexity of attacks that often span multiple domains and organizations, necessitating a unified response.
The emphasis on enhanced intrusion detection systems illustrates another pivotal strength of this framework. Utilizing advanced techniques like graph neural networks (GNNs) and long short-term memory (LSTM) models, researchers have developed methods that excel at identifying malicious activities within network traffic. These tools contribute to a more accurate understanding of data flow patterns, enabling quicker responses to threats as they emerge. In an era where encrypted traffic is ubiquitous, such advancements are vital for maintaining visibility and security across networks.
The methodology employed in this research is equally compelling. By leveraging semi-supervised learning and novel approaches like traffic hypergraph learning, the study aims to bridge the knowledge gap that often hinders traditional security measures. The construction of hypergraphs allows for a nuanced representation of complex relationships between traffic flows, enhancing feature extraction processes for anomaly detection. This approach not only improves detection rates but also minimizes false positives—a perennial challenge in cybersecurity.
The findings also extend into practical realms with implications for IoT security management and adaptive security policies using reinforcement learning. As devices proliferate across various sectors, securing Internet of Things (IoT) ecosystems becomes imperative. FlowID’s capabilities can be tailored to monitor IoT environments, ensuring that vulnerabilities are addressed proactively rather than reactively. Furthermore, by implementing adaptive security policies informed by real-time data analysis, organizations can dynamically adjust their defenses against evolving threats.
While the strengths of FlowID are considerable, it is essential to acknowledge its limitations and areas ripe for further investigation. For instance, while the framework excels at detecting anomalies within specific contexts, cross-domain security applications remain an area requiring additional focus. Developing effective strategies for applying these techniques across diverse organizational boundaries will be key to maximizing their impact.
As we look ahead, the implications of this research signal a promising future for cybersecurity methodologies. The demand for continuous innovation is clear; as cyber threats grow more sophisticated and pervasive, so too must our defenses evolve. With frameworks like FlowID paving the way for automated threat intelligence generation and improved malware detection and classification, the prospects for achieving a more secure digital landscape are both exciting and essential. The journey toward comprehensive cybersecurity solutions is ongoing, but with concerted effort and collaboration, we may very well be on the brink of significant breakthroughs in protecting our interconnected world.