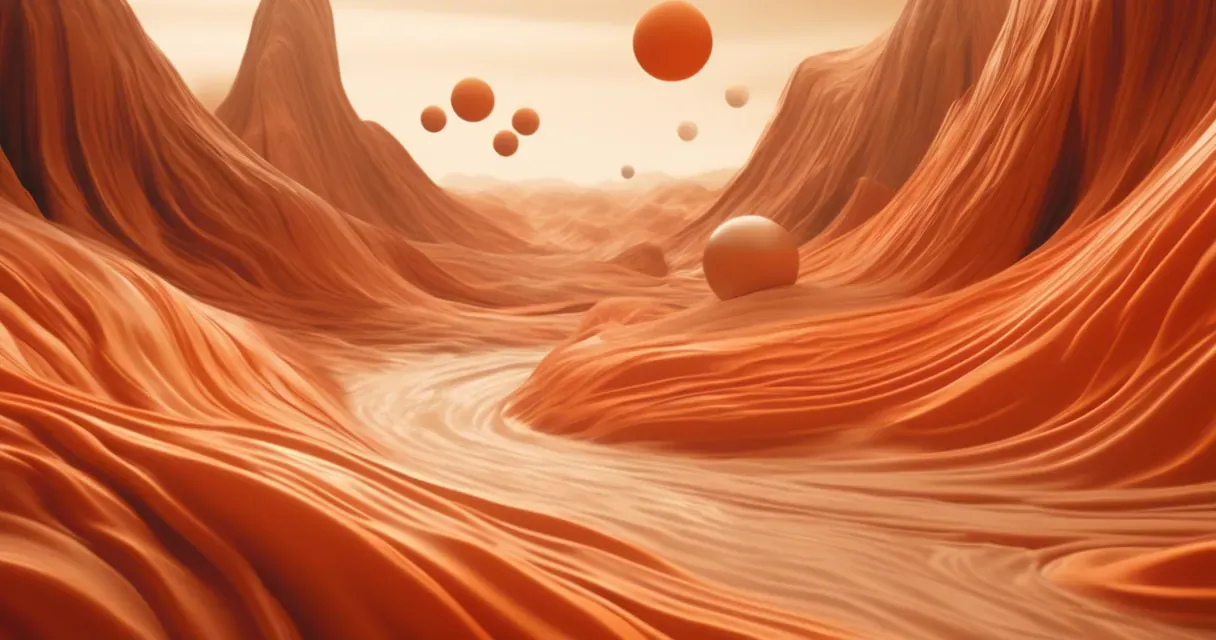
Meta-UAD: New Scheme for Network Traffic Anomaly Detection
/ 4 min read
Quick take - Researchers Xu and Zhang have introduced a novel approach to magnetic anomaly detection in cybersecurity using an attention-bi-directional Long Short-Term Memory network, aiming to improve anomaly detection in user-level network traffic and enhance security for Internet of Things devices and cyber-physical systems.
Fast Facts
- Researchers Xu and Zhang developed a novel magnetic anomaly detection (MAD) model using an attention-bi-directional Long Short-Term Memory (Bi-LSTM) network to enhance cybersecurity in user-level network traffic.
- The study employed a meta-learning framework (Meta-UAD) and conducted extensive experiments to validate the model’s effectiveness against existing detection techniques.
- Key findings include improved anomaly detection capabilities through an attention mechanism and the incorporation of federated learning for collaborative detection while maintaining data privacy.
- The research highlights the potential for advanced machine learning techniques to strengthen cybersecurity defenses across various domains, including IoT and cyber-physical systems.
- Limitations include the model’s computational complexity, prompting future research into optimization techniques and integration with threat intelligence platforms for enhanced security.
In an era where cyber threats evolve at a breakneck pace, researchers are continuously striving to develop innovative techniques that not only keep up with these advancements but also enhance the security framework of networks. One such promising development is Meta-UAD, a meta-learning scheme specifically designed for user-level network traffic anomaly detection. This groundbreaking research by Xu and Zhang showcases how machine learning can be harnessed to bolster network security through effective anomaly detection, offering a glimpse into the future of cybersecurity.
The backbone of this study lies in its attention mechanism, which plays a pivotal role in improving the model’s accuracy and reliability. By employing advanced deep learning techniques such as Bi-directional Long Short-Term Memory (Bi-LSTM) networks, Meta-UAD is able to intelligently focus on critical features in the dataset, leading to enhanced performance when compared to traditional methods. The authors evaluated the model’s effectiveness through rigorous experiments that highlighted its superior capabilities against existing detection techniques across various scenarios. These comprehensive evaluations serve as a testament to the potential of Meta-UAD in real-world applications, particularly in environments laden with diverse user traffic patterns.
One of the standout strengths of this research is its integration of federated learning. This collaborative approach not only enhances anomaly detection capabilities but also addresses privacy concerns prevalent in modern cybersecurity frameworks. By allowing multiple entities to train their models collaboratively without sharing sensitive data, federated learning ensures that organizations can collectively fortify their defenses while maintaining stringent data privacy standards.
As we delve deeper into the implications of this research, it becomes clear that there are numerous avenues for application, particularly in Internet of Things (IoT) device security. With billions of IoT devices connecting daily, ensuring their integrity is paramount. The proposed model could revolutionize how we monitor real-time anomalies within these networks, potentially catching threats before they escalate into full-blown attacks. Furthermore, integrating Meta-UAD with existing threat intelligence platforms could provide a holistic view of emerging threats, allowing organizations to stay one step ahead in their cybersecurity efforts.
Yet, despite its promising capabilities, the study does not shy away from acknowledging certain limitations. The computational complexity associated with training and inference may hinder the model’s applicability in resource-constrained environments or real-time applications. This aspect raises questions about scalability and the feasibility of deploying such advanced models across diverse organizational infrastructures.
In terms of future directions, several recommendations emerge from Xu and Zhang’s research. There is significant potential for exploring cross-domain anomaly detection solutions that leverage insights from various sectors—such as physical security monitoring and cyber-physical systems protection—to create robust defense mechanisms adaptable to multifaceted attack vectors. Additionally, enhancing feature extraction and selection processes could further augment the effectiveness of Meta-UAD in detecting sophisticated threats.
The findings presented not only highlight the immediate benefits of implementing advanced machine learning techniques in cybersecurity frameworks but also signal a transformative shift towards more intelligent and adaptive security solutions. As organizations increasingly recognize the importance of cybersecurity resilience, tools like Meta-UAD may very well become integral components of their defensive arsenals.
Looking forward, the integration of these advanced methodologies into everyday cybersecurity practices will likely redefine our approach to network protection. As researchers continue to refine these models and address existing limitations, we can anticipate a future where anomaly detection is not just reactive but predictive—empowering organizations to thwart potential threats before they materialize into tangible risks.